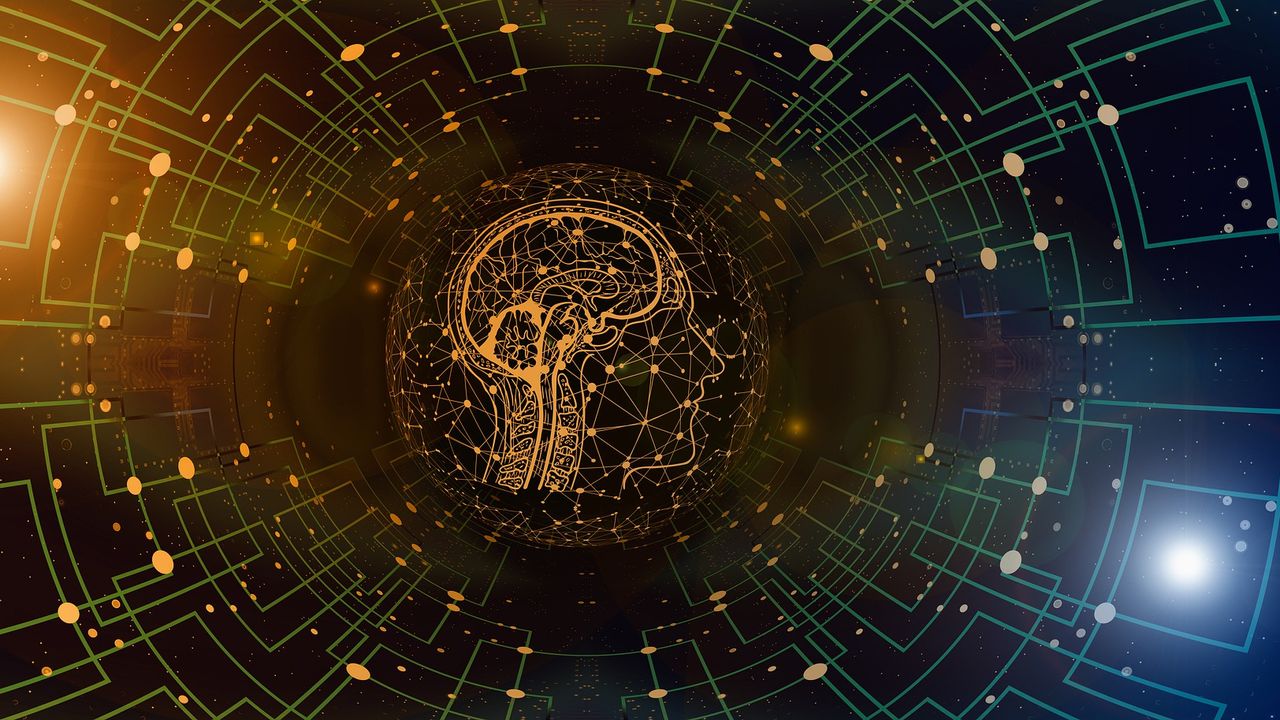
汽车需要定期检查,为什么AI型号有所不同?
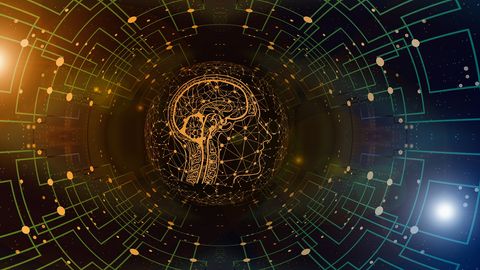
汽车需要定期检查,为什么AI型号有所不同?
填写下面的表格,我们将向您发送PDF版本的电子邮件"Cars Require Regular Inspection, Why Should AI Models Be any Different?"
This article includes research findings that are yet to be peer-reviewed. Results are therefore regarded as preliminary and should be interpreted as such. Find out about the role of the peer review process in researchhere。有关更多信息,请联系引用的来源。
It is taken for granted that cars require regular inspection and maintenance to ensure safety and reliability. On the other hand, with the intensified demand on digital transformation, many domains and industries are actively adopting artificial intelligence (AI) and machine learning (ML) for assisting decisioning making – ranging from autonomous vehicles, education, hiring, judiciary, health, trading, content recommendation and delivery, machine translation and summary, search and planning, interactive questioning and answering, robots, to scientific discovery, to name a few. But one critical question to reflect upon is:are we paying enough efforts, as seriously as to our cars, to inspect and certify the trustworthiness of these underlying AI-based systems and algorithms?此外,作为最终用户和消费者,我们真的知道AI技术如何以及为什么制定决策,以及AI技术在对抗性攻击方面的鲁棒性吗?
According to a recent Gartner report,130% of cyberattacks by 2022 will involve data poisoning, model theft or adversarial examples (see reference 2 for an overview of these new threats centered on machine learning). However, the industry seems underprepared. In a survey of 28 organizations spanning small as well as large organizations, 25 organizations did not know how to secure their AI/ML systems.3
There are many key factors associated with trustworthy AI, including fairness, explainability, privacy, transparency and robustness. In robustness, cars and trustworthy AI models share many common objectives. In what follows, we will highlight three analogies in car model development to explain why robustness is essential to AI models.
模型开发和部署的生命周期
Like the development of a car model (say, electrical cars), developing AI models is a costly and time-consuming process. The lifecycle of an AI model can be divided into two phases:训练anddeployment。培训阶段包括数据收集和预处理,模型选择(例如,体系结构搜索和设计),超参数调整,模型参数优化和验证。AI模型培训可能非常昂贵,尤其是在基础模型的培训方面4这需要在大规模数据集上进行预训练,该数据集具有由可训练的参数大小组成的神经网络。采用生成的预训练变压器3(GPT-3)5as an example, which is one of the largest languages models ever trained to date. GPT-3 has 175 billion parameters and is trained on a dataset consisting of 499 billion tokens. The estimated training cost is about 4.6 million US dollars even with the lowest priced GPU cloud on the market in 2020.6After model training, the model is “frozen” (fixed model architecture and parameters) and is ready for deployment. The two phases can be recurrent – a deployed model can reenter the training phase with continuous model/data updates. Having invested so much, one would expect the resulting AI model is hack-proof and robust to be deployed. Otherwise, the failure of an AI technology could be as catastrophic as car model recalls.
Error inspection and fault diagnosis in the lifecycle
When cars are in motion, there are several sensors in place for fault detection. During the AI model’s lifecycle, understanding the failure modes and limitations of the model can help model developers identify hidden risks and errors, and more importantly, mitigate negative impacts and damage before deployment in the real world. Depending on the assumption on the attackers’ capabilities in intervening the AI lifecycle, also known as the threat models, different attacks targeting ML-based systems are summarized in Figure 1.

Figure 1。Holistic view of adversarial attack categories and capabilities (threat models) in the training and deployment phases. In the deployment phase, the target (victim) can be an access-limited black-box system (e.g., a prediction API) or a transparent white-box model. Image adapted from Chen PY, Liu S. Holistic adversarial robustness of deep learning models. arXiv. doi:10.48550/arXiv.2202。
A thorough robustness inspection based on a comprehensive set of active in-house testing, continuous monitoring, and performance certification (e.g., quantifiable measure of robustness7)应将其视为AI技术的必备标准,以确保其安全性和可靠性。许多开源库,例如对抗性鲁棒性3608provide available tools for error inspection and robustness evaluation on machine learning models. As illustrated in Figure 2, upon the diagnosis, one can fix the identified issues and return a risk-mitigated model for use, just like the procedure of car inspection and maintenance!

Figure
2
。Conceptual pipeline for AI model inspection. The first stage is to identify any potential threats hidden in a given AI model. The second stage is to fix the found errors and eventually return a risk-mitigated model for use. Image adapted
fromhttps://youtu.be/rrqi86vqiuc。
Improving robustness in unseen and adversarial environments
像火星勘探流浪者这样的汽车可以在新的和看不见的地形上成功执行分配的任务,因为它们是在模拟环境上开发的。对于AI模型,可以合并从错误检查工具中产生的故障示例,以改善看不见甚至对抗环境中的鲁棒性。这种模型培训方法被称为adversarial machine learning,通过在训练环境中引入虚拟对手,以刺激更好,更健壮的模型。在模型训练期间,虚拟对手的作用是模拟最坏的情况,并生成新的数据样本,以帮助模型在看不见和对抗性环境中更好地推广。图3总结了这种新的学习范式的主要目标,包括发现限制,改善鲁棒性,创造协同作用和增强机器学习。值得注意的是,对抗机器学习还激发了许多新颖的应用程序,超出了鲁棒性的最初目标,例如模型重编程,该模型可提供有效的方法来重用预先训练的AI模型,以解决资源限时域中的新任务。9

Figure
3
。The methodology of learning with an adversary, also known as adversarial machine learning.
Cars are transformative technology and have deep influence on our society and life. However, we also need to acknowledge and address their accompanied issues such as energy consumption and air pollution. Similarly, while we are anticipating AI technology to bring fundamental and revolutionary changes, we need to be proactive to prepare our technology to be hack-proof and trustworthy.10AI鲁棒性研究的目标是在AI技术,社会和以人为中心的信任之间建立有机生态系统。如图2所示,可以通过形式化和制定AI模型检查的标准来实现这种生态系统,以确保更大的利益,防止可能的滥用,并快速适应自我识别的模拟失败以及野外的真实和不可预见的挑战。
参考
1。Gartner top 10 strategic technology trends for 2020. Gartner.https://www.gartner.com/smarterwithgartner/gartner-top-10-strategic-technology-trends-for-2020。于2019年10月21日发布。访问于2022年3月7日。
2。Chen PY, Liu S. Holistic adversarial robustness of deep learning models.arxiv。Posted online February 15, 2022. doi:10.48550/arXiv.2202
3. Kumar RSS,NyströmM,Lambert J等。对抗机器学习 - 行业观点。arxiv。Posted online February 4, 2020. doi:10.48550/arxiv.2002.05646
4. Bommasani R,Hudson DA,Adeli E等。关于基础模型的机会和风险。arxiv。Posted online August 16, 2021. doi: 10.48550/ARXIV.2108.07258
5。Brown TB, Mann B, Ryder N, et al. Language models are few-shot learners.arxiv。在线发布于2020年5月28日。Doi:10.48550/arxiv.2005.14165
6。Openai’s GPT-3 language model: a technical overview. Lambda.https://lambdalabs.com/blog/demystifying-gpt-3/。于2020年6月3日出版。2022年3月7日访问。
7。Preparing deep learning for the real world – on a wide scale. IBM Research.https://research.ibm.com/blog/deep-learning-real-world。Published February 9, 2021. Accessed March 7, 2022.
8.对抗性鲁棒性360. IBM研究。https://art360.mybluemix.net/。2022年3月7日访问。
9。Chen PY. Model reprogramming: resource-efficient cross-domain machine learning.arxiv。doi:10.48550/ARXIV.2202.10629。Posted online February 22, 2022.
10. Securing AI systems with adversarial robustness. IBM Research.https://research.ibm.com/blog/securing-ai-workflows-with-adversarial-robustness。Published February 9, 2021. Accessed March 7, 2022.